AI in Biotech: Will the Future of Science Favor Dry Labs Over Wet Labs?
- Guru Singh
- Apr 17
- 12 min read
Updated: 2 days ago
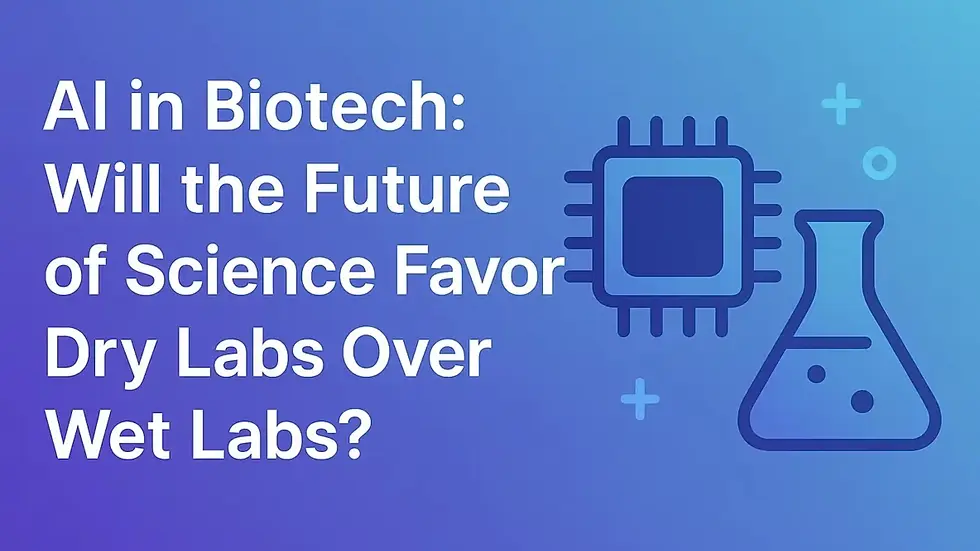
In biotech's push toward digitization, platforms like Scispot, offering the best AI stack to life science labs, are enabling a quiet revolution in how research is conducted. This transformation was a key theme in a recent episode of talk is biotech! where host Guru Singh, a biotechnology evangelist and product strategist, spoke with Kevin Chen, CEO of Hyasynth Bio, about the future of lab research. Singh suggests that advanced in silico testing (computer-driven experiments) could "slash errors, boost certainty, and shake up experiments". In his view, AI may significantly reduce the number of wet-lab experiments required, since many hypotheses can be tested digitally first. This raises a pivotal question for the industry: Will the labs of the future be dominated by computers and simulations (dry labs), and if so, what does that mean for traditional hands-on experimentation (wet labs)?
Dry Labs vs. Wet Labs in Biotech: Definitions and Context
Wet labs and dry labs represent two complementary sides of scientific research. A wet lab is a traditional laboratory space for hands-on experiments involving physical samples, whether mixing chemicals, culturing cells, or testing biological reactions. Wet labs deal with tangible materials (liquids, solids, gases) and are equipped with pipettes, Petri dishes, chemical fume hoods, and similar tools to safely handle these substances.
In contrast, a dry lab is a space dedicated to computational or theoretical work. Instead of test tubes and reagents, a dry lab features computers and software. Researchers in dry labs analyze data sets, build mathematical models, and run simulations to predict outcomes. A dry lab is one where "computational or applied mathematical analyses are done on a computer-generated model to simulate a phenomenon in the physical realm". In other words, dry labs allow scientists to experiment in silico (on the computer) rather than in vitro or in vivo (in the glass or in the living organism).
Historically, biotech and pharmaceutical R&D have been dominated by wet labs. Discoveries were driven by trial-and-error at the bench: biologists cloning genes, chemists mixing compounds, and pharmacologists testing drugs in petri dishes or animal models. This "pure experimental data over computer simulation" tradition has long defined the wet lab culture.
However, the rise of powerful computing and AI is changing that paradigm. The line between wet and dry labs is increasingly blurring. Today, many wet labs are augmented with computational tools for data analysis, while dry labs may closely guide experiments through simulations and data-driven predictions. The biotech lab of the 2020s is as likely to have machine learning algorithms crunching data as it is to have scientists pipetting at lab benches. This convergence sets the stage for reimagining how we conduct experiments.
The Rise of AI and In Silico Experimentation
Artificial intelligence is accelerating a shift toward "dry lab" approaches in biotech R&D, fundamentally reshaping the scientific process. Guru Singh, in his podcast conversation, highlighted that AI-driven in silico testing can dramatically increase the efficiency and certainty of research. By simulating experiments on a computer, scientists can identify promising hypotheses and eliminate dead ends before ever touching a physical sample. As Singh noted, AI might even make some wet lab work obsolete: why perform dozens of tedious bench experiments when a well-trained model can test those hypotheses virtually in minutes? "Will AI make wet labs a thing of the past or supercharge them instead?" he asks pointedly.
Real-world examples already illustrate this trend. For instance, drug discovery traditionally involved synthesizing and testing thousands of compounds in wet lab assays to find a viable drug candidate, a costly and time-consuming process. Today, AI algorithms can screen millions of virtual compounds against disease targets in silico, narrowing down a handful of promising candidates in a fraction of the time. Researchers can then take those top candidates into the wet lab for focused testing. This approach has proven effective: scientists can now explore "orders of magnitude more chemical structures" via AI than physical screening would allow.
Similarly, predicting a protein's 3D structure used to require laborious wet-lab techniques like X-ray crystallography; now, AI systems like DeepMind's AlphaFold can compute protein structures with remarkable accuracy on a computer. These digital breakthroughs don't eliminate the need for lab validation, but they massively reduce the experimental burden by pre-validating many ideas computationally.
Kevin Chen's own experience at Hyasynth Bio underscores how much can be achieved with minimal wet lab work early on. Hyasynth is a synthetic biology startup that engineers yeast to produce cannabinoids (compounds traditionally harvested from cannabis plants). In describing Hyasynth's "zero-to-one" journey, Guru Singh noted that the team started with "no lab, no gear, no playbook, just a wild idea". They relied on smart experimental design and leveraging existing knowledge (and likely some computational planning) before setting up any physical lab infrastructure. Only once their designs were sound did they hustle to actually engineer enzymes and run fermentation experiments to turn their idea into reality.
This story exemplifies a broader pattern: new biotech ventures can begin with heavy reliance on dry lab work, literature mining, genetic circuit design software, bioinformatics analyses, and postpone extensive wet lab work until later. AI is amplifying this capability by providing more sophisticated modeling and predictive analytics at the earliest stages of research.
From Singh's perspective, this trend means we'll see fewer total wet lab experiments in the future, because many will have been rendered unnecessary by accurate in silico results. Importantly, those wet lab experiments that are conducted can be more targeted and likely to succeed, since they've been pre-vetted by AI. It's a bit like aerospace engineers using computer simulations to test aircraft designs before ever entering a wind tunnel. By the time a physical prototype is built, the designers have high confidence it will fly. In biotech, if we can virtually test a new drug on a simulated cell or run thousands of virtual gene edits to see which yields the desired outcome, the handful of real experiments we perform afterwards are far more likely to work. This reduces costly trial-and-error and speeds up innovation.
Strengths of the Dry Lab Approach
Why are AI-powered dry labs so attractive? There are several key advantages to conducting experiments in silico before (or even instead of) at the bench:
Speed and Scale: Computational experiments can be run quickly and in parallel. A computer cluster can simulate thousands of conditions or molecular designs overnight, something that would take months or years in a wet lab. AI models can rapidly sift through vast solution spaces: for example, a machine learning model can evaluate millions of drug-like molecules in silico, whereas a chemist might only be able to physically test a few hundred compounds. This dramatically accelerates the early research funnel, focusing resources on the most promising leads.
Cost Efficiency: Wet lab work is expensive. Reagents, lab equipment, and skilled technicians all cost money, and failed experiments add up. Dry lab work, by comparison, primarily incurs computing costs and salaries for data scientists (and cloud computing is relatively cheap for many tasks). By performing extensive hypothesis testing via AI and simulations first, companies can save significant time and money before committing to physical experiments. A dry lab analysis might reveal that half of a project's ideas won't pan out, sparing the team from spending resources on those dead ends in the wet lab.
Reduced Risk and Error: Working with pathogenic bacteria, toxic chemicals, or expensive clinical samples carries safety risks and the potential for irreproducible errors. In silico experiments involve none of these hazards. No one gets hurt by a computer simulation, and it won't "spill" a dangerous chemical. Moreover, AI-driven analyses can sometimes detect subtle patterns or optimal conditions that humans might miss, thereby designing experiments with higher likelihood of success. By the time a hypothesis moves to the wet lab, the variables are better understood and the experiment can be more precisely executed, potentially reducing human error or the need for repeated trials.
Exploring the Impossible or Complex: Some scientific questions simply can't be answered easily with direct wet-lab experimentation. For example, modeling the event horizon of a black hole or simulating how a protein folds over milliseconds. Dry labs shine in tackling such problems through mathematical modeling and simulation. In biology, researchers can simulate evolutionary processes, predict how a protein might mutate over decades, or virtually screen drug effects on simulated organs, scenarios that would be impractical or unethical to test directly in living systems. AI has even enabled the concept of "digital twins" in biotech: a computer model of a cell or bioreactor that can be probed in ways you could never do to the real thing.
It's important to note that dry lab work often precedes wet lab work in modern R&D workflows. Analysis in a dry lab is usually done before a wet lab is necessary, to guide and streamline the subsequent hands-on development. In practice, a project might cycle through dry and wet phases: start with computer modeling to generate a hypothesis, use the model's predictions to plan a focused experiment in the wet lab, then feed the experimental results back into refining the model. This iterative loop can yield a powerful "virtuous cycle from lab to data and back to the lab", combining the strengths of both approaches.
Why Wet Labs Remain Indispensable
Given the impressive capabilities of AI and dry labs, one might wonder: will we even need wet labs in the future? The consensus among experts is a resounding yes. Wet labs are here to stay. While AI can model known data and suggest likely outcomes, biology always has elements of unpredictability and complexity that only real-world experiments can reveal. As one scientist noted, the notion that dry labs might entirely replace wet labs is "not plausible. Wet lab experiments are indispensable, as they provide empirical validation and uncover complex biological phenomena that cannot be fully captured by theoretical models or simulations". In other words, no matter how sophisticated our computer models become, we will always need to verify AI's predictions in actual cells, tissues, and organisms. Wet labs remain the ultimate proving ground for scientific truth.
AI predictions are based on existing datasets and learned patterns; they can sometimes be biased or limited by the quality of data available. Wet lab experiments, by contrast, can discover new phenomena and surprise us. History is full of serendipitous wet-lab discoveries. Penicillin's discovery from a mold contamination, or CRISPR gene editing found in a bacterial immune mechanism, are breakthroughs that weren't predicted by any model. By doing physical experiments, researchers can observe effects that no algorithm anticipated. This creative, exploratory aspect of wet lab science is hard to replicate in silico.
Moreover, biological systems are incredibly complex and context-dependent. A drug that looks perfect in a computer model might fail in a real organism due to metabolism or off-target effects; a gene circuit simulated in software might behave unpredictably in an actual cell. "Dry lab tools generate theoretical results... their predictions often require validation through in vitro and in vivo experiments", cautions Bhaskar Chatterjee of Razi University. He emphasizes that while dry labs are invaluable for narrowing down hypotheses, wet labs remain the cornerstone of discovery, providing ground truth about how living systems actually behave.
In practice, the best results come when we treat dry and wet methods as partners rather than competitors. The data from wet labs can refine AI models, and AI models can suggest better experiments for the wet lab, a complementary cycle rather than a zero-sum replacement.
Another reason wet labs will remain critical is the need for regulatory and empirical proof. In biotechnology industries (pharma, medical devices, agriculture), regulatory agencies require hard evidence from wet-lab experiments and clinical trials to approve new products. A computational prediction, no matter how advanced, is usually not sufficient to meet safety and efficacy standards. For example, an AI might predict a drug is non-toxic, but only lab and animal tests can confirm that with confidence. Thus, wet labs are integral not just for discovery but also for validation and compliance.
The Future of R&D: Integrating AI, Dry Labs, and Wet Labs
Rather than AI completely shifting science to one side (dry) or the other (wet), the future of biotech R&D is likely to integrate both approaches even more deeply. Guru Singh's question, will AI make wet labs obsolete or supercharge them, hints at the answer. The most probable scenario is that AI will supercharge wet labs, making them smarter, more efficient, and more accessible, rather than rendering them irrelevant.
We are already seeing the advent of "smart labs" where automation and AI planning are embedded in the experimental workflow. In such labs, robotics handle routine wet-lab tasks while AI algorithms plan experiments and analyze data in real-time, creating a tight feedback loop between the physical and digital realms.
In the podcast, Singh and Chen also touch on how these trends could democratize who can do biotech research. As lab processes become more digitized and standardized, "wet labs might soon be as plug-and-play as Lego kits", Singh wrote in a promo for the episode. This alludes to a future where setting up a biology experiment could be as straightforward as snapping together modular components, guided by AI, lowering the barrier for new entrants.
Indeed, Chen discussed the rise of citizen science and DIY biology. With AI and better tools, biotech is "starting to feel less like sterile institutions and more like creative playgrounds" open to a broader community. One can imagine cloud-based dry labs and contract wet lab facilities enabling even small startups (or hobbyists) to design experiments digitally and then execute them via automated services. The upshot: the future of science may not favor dry over wet, but rather fuse them, allowing anyone with a good idea to leverage both AI and physical experimentation without massive infrastructure.
In Chen's words, the future of biotech could belong to everyone, not just PhDs in big labs, as AI lowers the expertise and cost hurdles for innovation.
Technologically, the integration is happening on multiple fronts. Lab software platforms (for example, Scispot's LabOps tools) are being built to connect wet and dry workflows, ensuring data flows seamlessly from experimental apparatus to analysis algorithms. Companies are increasingly hiring hybrid scientists, people comfortable with both bench science and coding, to act as the bridge between wet and dry teams. And investors are pouring capital into AI-driven biotech startups on the premise that they can iterate faster and succeed with fewer costly lab failures.
In short, the labs of the future will be "AI-augmented": not dry vs. wet, but a continuum where computational models guide physical experiments, and those experiment results in turn improve the models.
Strategic Implications for Biotech Firms
For biotechnology companies and research organizations navigating this transition toward AI-powered research, several strategic implications emerge:
Adopt an AI-First, Experiment-Second Mindset: Organizations should integrate AI and computational modeling early in their R&D process. By testing ideas in silico first, firms can prioritize the most promising experiments to run in wet labs. This approach can shorten development cycles and cut costs by reducing the number of failed bench experiments. Companies like Insilico Medicine have already demonstrated AI-designed drug candidates that moved to trials much faster than traditional methods, illustrating the competitive edge an AI-first strategy can provide.
Invest in Digital Infrastructure and Data Integration: A key requirement for effective dry lab work is high-quality data. Biotech firms must ensure their wet-lab experiments are generating reliable, well-annotated data that AI tools can learn from. This may involve upgrading laboratory information management systems (LIMS), electronic lab notebooks, and data storage to be AI-ready. Platforms that unify wet and dry lab data (such as lab management software with AI integration) can break down silos and enable the virtuous cycle of model refinement and experiment planning. Firms should treat data as a strategic asset and continuously feed their AI models with experimental results (both successes and failures) to improve predictions over time.
Develop Cross-Functional Talent and Collaboration: The most successful biotech teams will blend wet-lab expertise with computational savvy. Companies should foster collaboration between bench scientists and data scientists, and even consider training programs to upskill traditional biologists in coding or machine learning. Conversely, computational biologists should be encouraged to understand experimental design and context. Bridging the culture gap is important. As Scispot's analysis highlights, differing "tools, languages, and methodologies" between wet and dry teams can cause friction if not addressed. Some firms are creating new roles (e.g., automation scientist, computational chemist, AI lab lead) that explicitly straddle both worlds. A culture that encourages open communication between modelers and experimentalists will be more productive and innovative.
Balance AI Enthusiasm with Empirical Rigor: While it's tempting to lean heavily on AI, firms must remember that physical validation is paramount. It is prudent to view AI as an aid to, not a substitute for, experimental science. For every computational result that guides a project, a clear plan should exist for how it will be confirmed in the wet lab or in vivo. This balanced approach guards against overconfidence in silico predictions and ensures that products and discoveries ultimately rest on solid empirical evidence. In practice, this means maintaining strong wet lab capabilities (in-house or through partners) even as you expand your dry lab toolkit. Regulators, investors, and patients will trust outcomes that have been validated in the real world, so companies should use AI to funnel their wet-lab efforts, not to bypass them.
Leverage AI to "Supercharge" Your Wet Labs: Rather than viewing AI and automation as making human scientists obsolete, forward-looking organizations use these tools to enhance their researchers' productivity. Automating routine lab tasks (through robotics or software) frees scientists to focus on design and interpretation. AI can suggest experiment optimizations (e.g., optimal gene editing targets or best compound dosages) that scientists might not have considered, functioning like a smart assistant. By embracing such technology, even smaller labs can perform at a level that previously required far greater manpower and resources. The goal should be a wet-dry symbiosis: let the AI handle data crunching and pattern recognition at scale, while human experts apply creativity and judgment to decide which experiments to run and to make sense of the outcomes.
Conclusion
In conclusion, the future of science will not be an either-or choice between dry labs and wet labs, but rather a melded ecosystem that exploits the best of both. AI is tilting the balance toward more in silico work upfront, favoring dry labs for hypothesis generation and predictive modeling, but this doesn't diminish the importance of wet labs. Instead, it elevates the role of wet labs to a validation and discovery engine for the most critical questions, guided by digital insight. The labs of tomorrow are poised to be more efficient, creative, and inclusive, as AI-driven dry lab tools converge with automated, plug-and-play wet lab platforms. Biotech leaders would do well to ride this wave: those who integrate AI thoughtfully into their R&D pipelines will likely leap ahead, while those who ignore it may find themselves stuck pipetting in the slow lane. As Guru Singh's discussion with Kevin Chen illustrates, embracing this AI-powered evolution isn't just about adopting new tech, it's about reimagining the scientific method itself, so that bits and bytes work hand-in-hand with test tubes and pipettes in the service of discovery.
Commentaires